Can human centric creative process be improved by predictions?
Sinead O'Brien
Lead Project Manager, TS&A
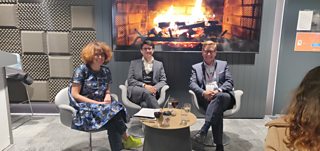
On Wednesday May 1st, I attended the latest edition of the Βι¶ΉΤΌΕΔ Machine Learning Fireside Chat series on predictions and the human centric creative process.
“This time we will explore the state of the art of collaboration between machine generated predictions and people in the creative process. What is the work ahead of us in order to make this joint venture better? What is going to be the role of increased interpretability of predictions, the role of interfaces and how the work of content creator is going to change? Finally what are the dangers, and consequences to the business and the audience?”
The panel hosted by Magda Piatkowska, Head of Data Science Solutions at Βι¶ΉΤΌΕΔ News, included Greg Detre, Chief Data Scientist at Channel 4, and Atte Jääskeläinen, a renowned ML researcher and former Director of News and Current Affairs at Finnish station YLE, considered to be a leading innovator among public service broadcasters.
The panel kicked off with a direct question. Are we fearful about how machines will destroy creativity and replace journalists?
Atte shared his view that there is indeed fear. Different organisations have different communications and leadership attitudes, which influence whether the landscape is presented as a threat or an opportunity. The nature of work will change and we need to question how creative work can be more productive, interesting and creative. Greg pointed to Aristotle and 19th century Luddites to articulate the existence of a long term considerable fear.
ML Tech as Enabler or Disruptor?
Magda probed which stages of the newsroom process, from gathering to publishing, are most likely to be impacted. Atte pointed to the business models funding journalism, specifically examples of Facebook and Google who are targeting users in better ways than traditional news organisations. Greg expected change to take a long time, and reminded us that Deep Blue vs Kasparov happened in 1997, over twenty years ago.
Humans are in the driving seat, adding something to machine output. What will the interfaces look like? Greg expected this to be more like dialogue. At Channel 4, human experts use a combination of historical data and research for forecasting. By replacing the human process with machines, it will sometimes be better and sometimes worse. Humans understand warning signs and can interrogate and tweak accordingly. Atte noted that with media, people pay for negative feelings. Magda added that Public Service Media should remember that private media consider public interest as part of the optimisation of algorithms too.
Collaboration: Human and Machine?
Atte provided two examples. Firstly, the prediction of successful news stories; and second, for commercial news organisations, the prediction of how a digital news story contributes to a willingness to buy a digital subscription. But we need to manage expectations. The machine may not be able to predict as well as the newsroom thinks. Most news organisations are trying to find ways of being more interesting to younger audiences but we have an issue with identifying how we engage with younger people. Greg spoke of empowering editorial teams. Algorithmic recommendations are based on original ideas of human beings. So how do we make the machine’s response better? How do we build trust so that the machine will continuously do well for the audience?
We acknowledge that failure is not catastrophic in the context of TV recommendations, not like it could be in the case of AI driven cars. Atte contended that we need to make it clear that things need to change and to create a positive environment where change is seen as a positive opportunity. News editors can be afforded more time for thinking. This can be a positive disruption. People can concentrate on doing things they are more interested in and in being more creative. Greg pointed out that hardware is evolving so much slower than software. He likened the current climate to a dancing bear. The bear may not dance well but there is general amazement that the bear can dance at all. If is good enough to generate fake news, it is good enough to be dangerous.
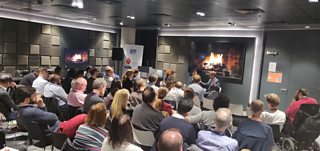
An audience member echoed the fact that most algorithms are goal seeking. Is there a danger that AI journalism will optimise for what is NOT best for society? Atte agreed that there is a danger. But stated that we must move forward and design good algorithms. Greg took the example of recommendations. What would someone who had watched everything and who knew you well recommend to you? Look at how an algorithm does this. It is possible to layer on a human component to interject.
Atte shared a tale of AI whisky. There are an infinite number of possibilities for raw whisky in barrels. The algorithm generates the ideas. However, to fit with the brand of the whisky house, there are limited proposals. So the final selection decision is made by the master blender. This first AI whisky is due to hit the shops in September! Atte’s example is about getting to the root of what is valuable.
Greg shared his definition of intelligence as “flexible, gold rated behaviour”. It is that flexibility that makes us interesting as human beings. The quality of journalism is a multidimensional issue according to Atte. Algorithms don’t grasp contextual understanding of what words mean, like humans do. Atte however maintained that algorithmic bias is easier to understand than human bias in a way. ML can help the newsroom in the way they think by providing a mirror.
From a commercial perspective, cheaper to produce is not always the answer. The real difference comes from where the real value is created, by a very small portion of the production. Today, as we measure consumption, we notice that only a small portion of articles are consumed. Do we want better content or more content? Greg is sceptical whether AI has anything to contribute here. We need to consider focus; where we can make an impact rather than trying to do everything. Atte added that it is hard to compete with the giants with large amounts of data and the costs of competing are high. Products being developed for other industries can be applied in media however.
Audience Discussion
Greg told us that it is possible to craft images that can trick the algorithm into thinking it is something else. It exists and will get more subtle and we should be worried about this. Atte said this reminds him of when people didn't know what bots were. The fake news problem is not about ‘do you trust this piece of news?’ but rather ‘do you trust this news organisation?’.
How do we measure trust and reflect that in how we build it into the system? An audience member stated that looking for a particular journalist is in actual fact searching for a particular bias, and so this is no different to ML in his view. Atte’s take is a little different as he believes it’s down to whether you share the values of those who tell you the stories. In the UK there are a number of political views shared through the newspapers. At Channel 4, everything consequential is checked by humans. Greg added that he thinks human concern will fade when machines become increasingly ‘right’. But they will still sometimes get it wrong. There is a problem of societal trust in media in parts of the world where media isn’t trustworthy.
The criteria for a successful news story?
There are more and more ways to engage people to pay a subscription and to stay as subscribers. Atte questioned “what should be the indicators of success?”. The answer is in the the value that content is creating for our private life and for society. The Βι¶ΉΤΌΕΔ is working heavily in this area. The European Broadcasting Union will also be focusing more on this next year.
Madga sees it as a numeric and simple equation, whereby success is increased page views shared across news sites. Content, for example, that stimulates public discourse such as the environmental conversation from Blue Planet. This was a story of huge impact story prompted both public conversation and action. However this is anecdotal and difficult to measure. ‘Impact’ as a metric is a difficult one. One person’s positive can be another person’s negative and if you define impact yourself, it is your measure. Atte added that monetary bonuses negatively impact the quality of work, thinking and creativity as we can’t know in advance what the most successful solution will be.
A human connection to the audience must be maintained. People are appreciating better selections of content. A solid voice can only be created by humans working alongside machines. Atte maintained that organisations will have to specialise more, to take care of one repertoire of the audience and to be the best. When the machine is right and we don’t take the recommendation, we overlook the better decision.
Greg picked up on how our relationship as designers of complex adaptive systems will change. We think of ourselves as controllers / programmers but really the role is more one of guidance, like an orchestra conductor. The relationship will need to evolve; our relationships with machines will change. Atte closed on the point that we tend to reject ideas that go against our human biases. But machines create ideas from which we can choose. There is a cultural change required in thinking that someone can have a better idea than you.