As part of our collaborative research work, ΒιΆΉΤΌΕΔ R&D sponsors a number of PhD students. These researchers work closely with ΒιΆΉΤΌΕΔ teams in all sorts of relevant fields, and many different universities. In this blog post our student from the University of Edinburgh Auste Simkute writes about her work on this with ΒιΆΉΤΌΕΔ R&D as part of our Data Science Research Partnership.
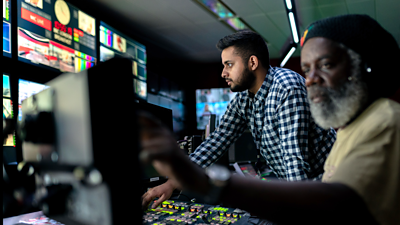
If you have ever applied for a credit card, searched for an image online, or used any software to translate a text, Machine Learning (ML) was probably involved in the process. However, ML models are only as good as the data used to train them. Without a meaningful human oversight historical biases in training data can even lead to discrimination and perpetuate social inequalities. For example, Amazon eventually disposed of their notorious hiring algorithm, which prioritised men instead of women as it was trained on the historic data of mainly men applicants ().
Similarly, ML tools used for image recognition have been shown to be much more accurate identifying white male faces, than black female faces. Even software sold by leading tech companies misclassified the faces of Oprah Winfrey, Michelle Obama, and Serena Williams (Bualamwini, 2019). Similar ML-driven tools are increasingly used by journalists and editors, for example, for selecting an image for a breaking news story from a vast database. Here the decision-maker needs to be able to judge if the image is not misclassified, and it is indeed of a person that it is expected to be.
Worryingly, biases in ML outputs are difficult to notice, and they often only become apparent after causing damage and discriminating against individuals or whole communities. Because of these risks, the argument is that there should be a human involved in the decision-making process, preferably a domain expert. However, simply telling an expert to supervise ML is insufficient (Green & Chen, 2019). Given how complex and opaque some ML models are, it would be naΓ―ve to expect experts to notice potential errors and ensure that ML is making fair and trustworthy decisions. In a media context, if news is to be balanced, fair and accurate, the expertise of the journalist needs to be able to be effectively utilised. If content is high quality, content producers need to be able to harness the power of tools that include automation but be able to use it to enhance not compromise the experience.
One of the solutions ML engineers and researchers have come up with is applying a set of techniques that explain either the model or a particular decision. This is often called Explainable Artificial Intelligence (XAI). However, technical XAI solutions lack usability and are ineffective when applied in domains where stakeholders have little or no ML expertise. In these cases users remain unable to make a justifiable decision. They either show automation bias and overtrust the prediction, or reject algorithmic decisions without considering them, expressing algorithmic aversion (De-Arteaga et al., 2020).
I argue that users who spend years making decisions without ML support, might struggle to apply their decision-making strategies in a completely different - algorithmic - context. Helping them to understand the logic behind the ML might not be enough to ensure they can use their expertise. I suggest that we should use design to embed these explanations into an interface that would support expertsβ reasoning.
In areas such as air traffic control, decision-makers were introduced to the complex decision-support technologies/automation long before ML became ubiquitous in other domains (Westin et al., 2013). They had to adapt, and one way their expertise was supported was by improving the interface design and making it work for the decision-making strategies and the way these experts reason. For example, interface features were designed to help air traffic controllers to reason on the locations, flight directions and proximities of neighbouring aircraft. Adding a simple cone of the triangle to illustrate the shape and orientations of the conflict zone to the interface made it easier for the expert controllers to link aircraft to their corresponding conflict zones. Experts reported then being able to draw an imaginary line from the aircraft blip toward the tip of the triangle - a reasoning strategy they were used to apply in their decisioning (Borst et al., 2017).
Similarly, by adding tailored interface features we could potentially support experts working with ML systems. Cognitive psychology and human factors literature can help to understand how experts reason and make decisions and what contextual factors moderate these processes. For example, we know that a highly experienced person could recognise unusual patterns in data and notice irregularities that might have potential implications with relative ease (Klein, 2003). This intuitive decision-making takes years of deliberate practice to develop and thus it is unique to experts. However, expert users need to see noise in data to use this valuable skill. Tailored explainability interface could, for example, enable this by providing an interactive manipulation of attribute values and observe how the output changes accordingly.
I recently wrote a paper for the Journal of Responsible Technology (Simkute et al., 2021), in which I connected decision-making strategies to the supporting design approaches and developed the Expertise, Risk, & Time (ERT) Framework. The suggested design framework is based on the psychology of human decision-making, especially that of experts. The underlying idea is that explanations could be embedded in an interface that would facilitate naturalistic decision-making. Interface designers should consider the context when developing explainability interfaces and ask three questions: How experienced is the decision-maker? How much time is available to complete this task? What are the consequences if there is an error? For example, a highly experienced ΒιΆΉΤΌΕΔ journalist writing a breaking news story on a sensitive topic would qualify for i) high expertise, ii) high time pressure, iii) high risk. The ERT framework should help designers match a given context to most suitable design strategies.
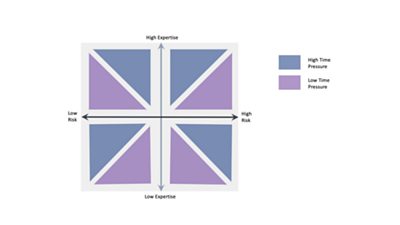
In the journal paper we used a scenario-based design approach to illustrate how designers might deploy the ERT framework. One scenario used an example of an experienced journalist Ada (high expertise) using a facial recognition and image quality classification tool to identify and choose the best images for a breaking news story (high time-pressure) about the meeting of political leaders for a G7 summit meeting. This is the dayβs top story that millions of people will read on the website homepage so any errors could cause reputational damage for the news organisation and herself (high risk).
As Ada types up her report about a meeting between UK Prime Minister Boris Johnson, US President Joe Biden and South African President Cyril Ramaphosa, the decision-support system identifies keywords to suggest a βtop 5β² selection of images based on relevance and quality of image. Ada rapidly scans the images β she is unsure what Ramaphosa looks like so she chooses the top-rated picture, of what she believes is the three men. Ada wishes she knew why the system was rating this one so highly and whether it is sure the third man is Ramaphosa, but there is no explanation provided. In this situation, an explainability interface should be tailored for high journalistic expertise, high time-pressure and high-risk context.
For this combination of factors the framework suggests moderating the amount of information provided, ensuring that it is presented sequentially, supporting mental simulation/imagery and reducing cognitive strain by visualising information. This suggests that including a visualisation which would gradually reveal features that were the most influential towards the ML image selection and ratings (e.g., based on feature importance) would be the most suitable approach in this situation.
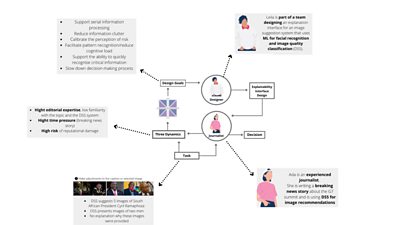
Artificial intelligence and machine learning plays an increasingly important role in the ΒιΆΉΤΌΕΔ, where algorithm-driven systems are used to automate mundane tasks, streamline processes, and deliver personalised services to customers. Together with the increased use of AI and automated systems, the ΒιΆΉΤΌΕΔ is also seeking to ensure that they are developed and applied responsibly and ethically. When used effectively these systems could improve production processes and support media experts in their day-to-day tasks. Journalists, editors, producers and other experts across the ΒιΆΉΤΌΕΔ could avoid spending time on tedious tasks and use it for valuable, creative elements of their roles.
My work is looking into how to make this human-machine collaboration responsible by enabling experts to harness benefits of the ML at the same time maintaining their agency. My proposed tailored explainability could help media experts to understand the logic of how one or another output was produced, challenge and inspect ML outputs, and make final decisions with confidence.
References
- Buolamwini, J. (2019, February 7). Time.
- Borst, C., Bijsterbosch, V. A., Van Paassen, M. M., & Mulder, M. (2017). Ecological interface design: supporting fault diagnosis of automated advice in a supervisory air traffic control task. Cognition, Technology & Work, 19(4), 545-560.
- Dastin, J. (2018, October 11). Reuters.
- De-Arteaga, M., Fogliato, R., & Chouldechova, A. (2020, April). A case for humans-in-the-loop: Decisions in the presence of erroneous algorithmic scores. In Proceedings of the 2020 CHI Conference on Human Factors in Computing Systems (pp. 1-12).
- Green, B., & Chen, Y. (2019). The principles and limits of algorithm-in-the-loop decision making. Proceedings of the ACM on Human-Computer Interaction, 3(CSCW), 1-24.
- Simkute, A., Luger, E., Jones, B., Evans, M., & Jones, R. (2021). Explainability for experts: A design framework for making algorithms supporting expert decisions more explainable. Journal of Responsible Technology, 7, 100017.
- Westin, C. A., Borst, C., & Hilburn, B. (2013). 17th International Symposium on Aviation Psychology, 530-535.