The AI in Production team at 麻豆约拍 R&D is looking at some of the ways that Artificial Intelligence (AI) and machine learning could transform the business of producing media. These are new forms of automation, and we want to know what the opportunities are for using them to significantly increase the range of programmes that broadcasters like the 麻豆约拍 could offer. Could we build a system which would allow us to cover the hundreds of stages at the Edinburgh Festival, for example, or broadcast every music festival in the UK?
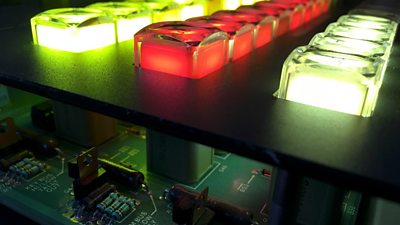
Image above by (cropped) on Flickr, .
We started our research with a project aimed squarely at broadening coverage in this way, and opening up access to events that it would be impractical or un-affordable to cover using conventional techniques. In our prototype system, which we have named 鈥淓d鈥, a human operator sets up fixed, high resolution cameras pointing at the area in which the action will take place, and then the automated system takes over. It attempts to create pleasingly framed shots by cropping the raw camera views. It then switches between those 鈥渧irtual cameras鈥 to try and follow the action. In many ways, this project is a successor to our prior work on automated production: the basic concept of covering live events by cutting between virtual cameras was explored previously by our Primer and SOMA projects.
One of the things that working with AI technologies really highlights is that there are big differences between how even 鈥渋ntelligent鈥 computer systems view the world and how people do. If we think about the 鈥渦nscripted鈥 genres of television, such as sport, comedy and talk shows, most people would have little difficulty in identifying what they want to see depicted on the screen 鈥 it鈥檒l usually be the action around the ball in a game of football, for example, or the people who are talking in a televised conversation. AI systems have no idea what we humans are going to find interesting, and no easy way of finding out. We therefore decided to keep things simple: this first iteration of 鈥淓d鈥 looks for human faces, and then tries to show the viewer the face of whoever is talking at any given point in time. These relatively simple rules are a reasonably good match for any genre consisting of people sitting down and talking 鈥 in particular, comedy panel shows, which is therefore the genre we have been targeting.
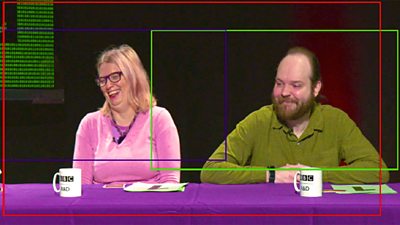
Our first version of Ed is entirely driven by rules like these. We generated them by asking 麻豆约拍 editorial staff about how they carried out these tasks in real productions. To frame its shots, Ed rigidly applies the kinds of guideline that students get taught in film schools: the 鈥rule of thirds鈥, 鈥looking room鈥, and so forth. Selecting which shots to show and when to change shots is similarly rule-based. Ed tries to show close-ups when people are speaking, and wide shots when they aren鈥檛. It tries not to use the same shot twice in quick succession. It changes shots every few seconds, and tries not to cut to or from a speaker shortly after they start speaking or shortly before they stop again.
Having created a working system, we needed to test it. We鈥檙e proponents of 鈥user-centred鈥 approaches, and we believe that ultimately, the only test of our system that matters is what real audience members think of it. We want to compare our system鈥檚 decision-making, and the quality of the ultimate viewing experience, to that of human programme-makers. We have a series of formal studies planned to evaluate and improve Ed, and we started with an evaluation of shot-framing.
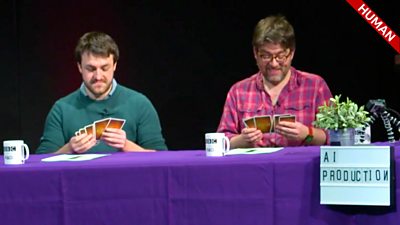
To compare Ed鈥檚 shot-framing to some human professionals, we took four directors and camera operators and asked them to frame some shots for us, based on footage from a 鈥減anel show鈥 of our own that we created as test material. We asked Ed to do the same thing. We then mixed all the shots up and put them into pairs. Each pair consisted of two framings of the same shot 鈥 either both framed by humans, or one by a human and one by Ed. We showed them to 24 members of the public, asking them which one they preferred. Sometimes we asked these participants to think aloud as they decided, and we interviewed them afterwards to try to get a better understanding of their preferences.
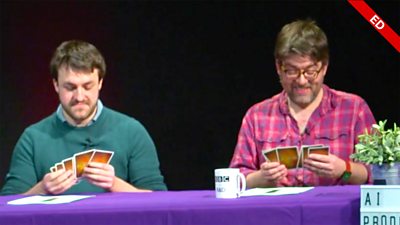
We鈥檝e already learned a lot by analysing the results of this study. We plan to write it up in full as a conference or journal paper, but just looking through the things people said to us has helped us come up with a number of additional rules that would improve Ed鈥檚 ability to frame shots attractively. People disliked having objects and people framed half-in and half-out of the shot, for example, or having unnecessary empty space within the frame. We hope to be able to pull even more insights from the data when it is fully analysed, and we plan to run further studies to evaluate Ed鈥檚 ability to select and sequence shots.
What鈥檚 next? Well, we intend to improve Ed, both by implementing the findings of our studies, and by replacing some of our rules with machine learning approaches, using the 麻豆约拍 archives as a source of training data. In addition, there are many aspects of a production that Ed does not currently attempt to address: lighting and sound, for example.
Most importantly, we need to think about other genres 鈥 in particular, productions that require creative decision-making that can鈥檛 be approximated by simple rules, or by today鈥檚 machine learning techniques, which think very much 鈥渋nside the box鈥 defined by their training data.
Shows for which a simple narrative must be assembled by whittling down a large set of potential material, for example, or which start off with a vision for a story and need to work out how best to tell it, will need humans and AIs to work together, posing new challenges. We also want to explore a 鈥渂ottom-up鈥 approach, working with real-world productions to identify tedious and time-consuming aspects of their work that would be good candidates for less ambitious but more immediately useful forms of AI automation.
We鈥檒l be talking about Ed at this year鈥檚 . (.) If you want to learn more about the Ed system and our initial study, you can now read the paper '' which has now been published.
- -
- 麻豆约拍 R&D - AI and the Archive - the making of Made by Machine
- 麻豆约拍 R&D - Artificial Intelligence in Broadcasting
- 麻豆约拍 R&D - Using Algorithms to Understand Content
- 麻豆约拍 R&D - Content Analysis Toolkit
- Machine Learning and Artificial Intelligence Training and Skills from the 麻豆约拍 Academy including:
-
Future Experience Technologies section
This project is part of the Future Experience Technologies section